fast.ai released part 2 of theirĀ Practical Deep Learning for CodersĀ free course. This one covers building a model like Stable Diffusion from scratch. Iām looking forward to going through it, since the foundations needed to implement the techniques are said to apply to many other types of models, but a more important lesson jumped out at me here.
Instead of starting with the foundations of transformers, attention, autoencoders, and so on, the course adopts a top-down method of learning. In this style you start by introducing the full solution to a problem, proceed from there to examine the component parts of the solution. If youāre ever been reading a book or taking a course, and have had trouble figuring outĀ whyĀ orĀ howĀ a particular chapterās content is useful, you can probably see why this style of learning would be helpful.
Starting with a full solution leaves some things unexplained or fuzzy at first, but I think it has a few advantages.
- Itās clear how new concepts fit into the bigger picture, because they can be introduced in the context of the initial, full example.
- Having a working example also helps with suggesting ways to explore the new concepts that might otherwise be presented in a vacuum, instead of just promising that it will all come together later.
- It makes it easier to jump right in and get something working right away, and for those who like to experiment, lets them start doing so more quickly.
Iāve been thinking about top-down learning so much because it applies directly to the spatial data book Iāve been writing.
After seeing how the fast.ai course is structured, this week I added a new chapter right at the beginning of the book. This new chapter is a working example that quickly introduces GeoJSON and D3 and presents a very simple web page that renders a map.
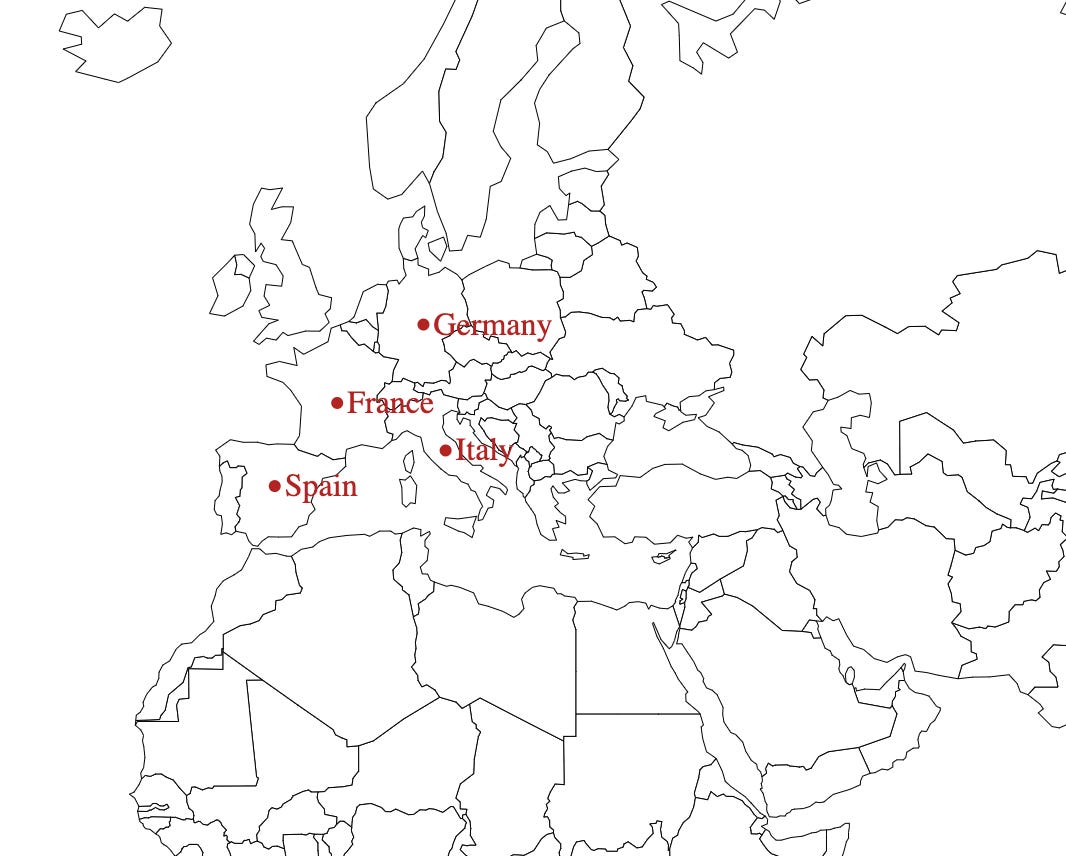
This map is nothing special ā just some country boundaries and a few points ā but serves a very important purpose by incorporating most of the concepts explored in more detail later in the book.
There are a lot of foundational concepts to cover when starting out with spatial data: the structure of GeoJSON, how to find and load spatial data into your application, and more. Now the reader can go into these foundational chapters with some idea of whatās actually going on, instead of having to wait until the last quarter of the book to make something they can actually see on the screen.
Aside from this new chapter, Iāve been updating the āTypes of Map Visualizationā chapter. This had been a very quick overview, but now Iāve been adding more examples and more pitfalls for each of the types of maps. The chapter will probably be three as long as it had been, but I expect that it will be much more useful and hopefully provide some guidance through what can be a tricky process even for seasoned data visualization practitioners.
Links
- Malleable software in the Age of LLMsĀ by Geoffrey Litt looks forward to how LLMs may bring software development to the masses, not so much in the sense of replacing existing software developers, but in making it much easier for non-technical people to create small one-off applications to solve specific applications. Worth some time to read and think about.